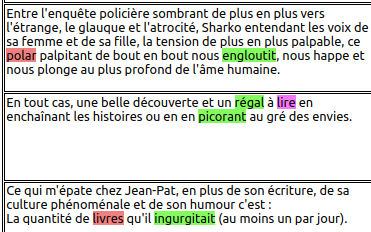
Babelio Food
A rule base model to detect food metaphor in Babelio comments (in French)
A rule base model to detect food metaphor in Babelio comments (in French)
The 2023 HackaTAL on Wikipedia and politics (in French)
A wrapper for the deepPavlov framework for Named Entity Recognition
A python package that aims to provide a basic API to create lexicons related to specific words.
A python package that scramble text using Unicode-looking-like characters.
The aim of this paper is to present the role of artificial intelligence and natural language processing techniques in the development of semi-automatic reading experience detection algorithms developed within the READ-IT project. Over the last decades, knowledge about the history of reading practices has increased considerably, but fundamental questions remain, such as "why" and "how" we read. By exploring digital sources for evidence of reading experiences, the READ-IT project (Reading Europe Advanced Data Investigation Tool, https://readit-project.eu) aims to better understand these phenomena.
The aim of this paper is to present an experiment of collaborative annotation of reading experiences carried out with students within the READ-IT project.
This paper describes a study on opinion analysis applied to both human to chatbot conversations, but also to human to human conversations using data coming from the banking sector. A polarity classifier model applied to conversations provides insights and visualisations of the satisfaction of users at a given time and its evolution. We conducted a study on the evolution of the opinion on the conversations started with the chatbot and then transferred to a human agent. This work illustrates how opinion analysis techniques can be applied to improve the user experience of the customers but also detect topics that generate frustrations with a chatbot or with human experts.
The information spread through the Web influences politics, stock markets, public health, people's reputation and brands. For these reasons, it is crucial to filter out false information. In this paper, we compare different automatic approaches for fake news detection based on statistical text analysis on the vaccination fake news dataset provided by the Storyzy company. Our CNN works better for discrimination of the larger classes (fake vs trusted) while the gradient boosting decision tree with feature stacking approach obtained better results for satire detection. We contribute by showing that efficient satire detection can be achieved using merged embeddings and a specific model, at the cost of larger classes. We also contribute by merging redundant information on purpose in order to better predict satire news from fake news and trusted news.